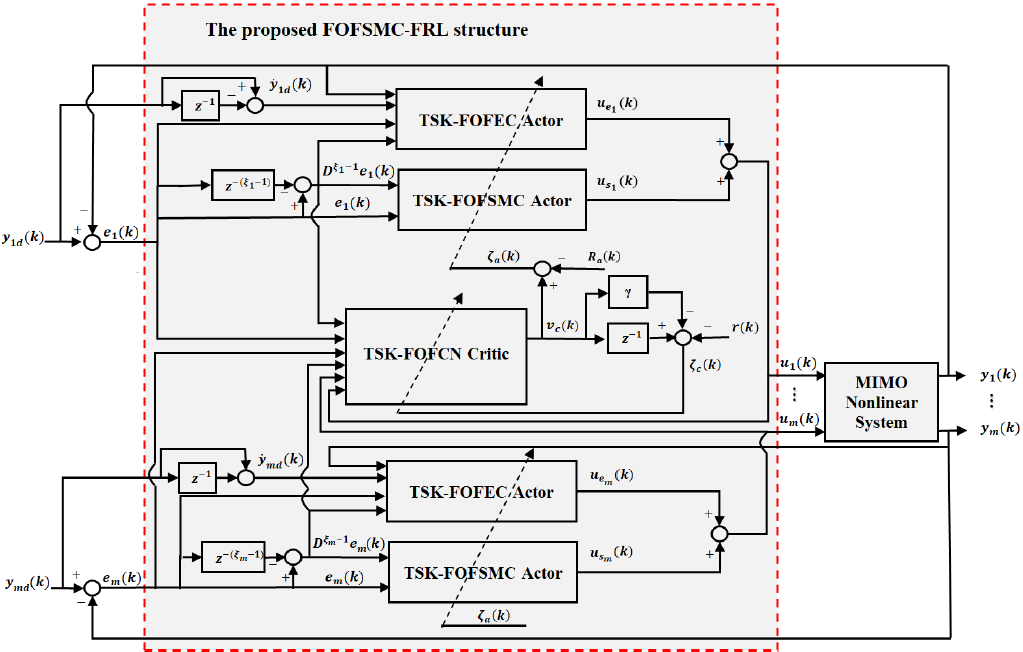
Fractional-order Fuzzy Sliding Mode Control of Uncertain Nonlinear MIMO Systems Using Fractional-order Reinforcement Learning
Abstract
This project presents a novel approach to enhance the control performance of unknown multiple-input and multiple-output (MIMO) nonlinear systems. The proposed method integrates a fractional-order fuzzy sliding mode controller with online fractional-order reinforcement learning (FOFSMC-FRL). The controller utilizes two Takagi–Sugeno–Kang (TSK) fuzzy neural network actors to approximate the equivalent and switch control parts of the sliding mode control, along with a TSK fuzzy neural network critic to estimate the value function for reinforcement learning. Parameters of the FOFSMC-FRL undergo online adaptation using a fractional-order Levenberg–Marquardt learning method (FOLM). The approach offers advantages such as adaptability to system dynamics, robustness against uncertainties, and elimination of the chattering phenomenon associated with sliding mode control. Comparative simulations demonstrate the superior performance of the proposed method over other control approaches, showcasing its efficacy in improving tracking accuracy and robustness against external disturbances.