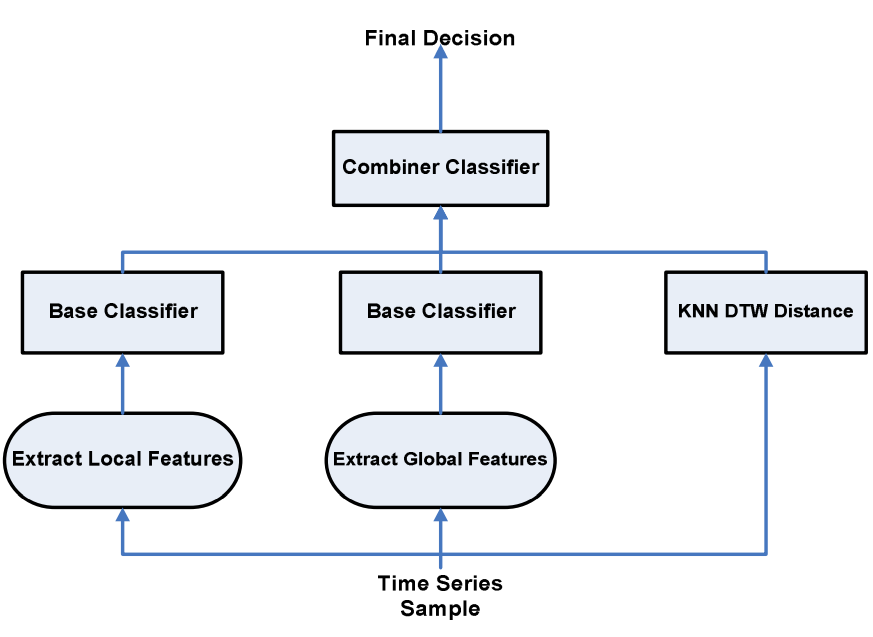
A time series classification approach for motion analysis using ensembles in Ubiquitous healthcare systems
Human motion analysis is a vital research area for healthcare systems. The increasing need for automated activity analysis inspired the design of low cost wireless sensors that can capture information under free living conditions. Body and Visual Sensor Networks can easily record human behavior within a home environment. In this paper we propose a multiple classifier system that uses time series data for human motion analysis. The proposed approach adaptively integrates feature extraction and distance based techniques for classifying impaired and normal walking gaits. Information from body sensors and multiple vision nodes are used to extract local and global features. Our proposed method is tested against various classifiers trained using different feature spaces. The results for the different training schemes are presented. We demonstrate that the proposed model outperforms the other presented classification methods. © Springer International Publishing Switzerland 2014.